Policies
Lakera Guard can be used to screen LLM interactions for a range of different threats. The configuration of the detector checks that will be run, the flagging logic, and their strictness are all controlled centrally via a Lakera Guard policy.
Through a policy, you can set up distinct configurations for each application, LLM-based feature, environment, or end user as you like. You can also configure and dynamically update individual apps and integrations’ Guard detectors and strictness on the fly to respond to threats or user experience issues, or to achieve your desired risk appetite.
Each project in Guard is assigned to a policy. Multiple projects can share the same policy, allowing you to manage defenses quickly and consistently across multiple applications, environments, features, and LLM integrations. A project cannot be assigned to multiple policies.
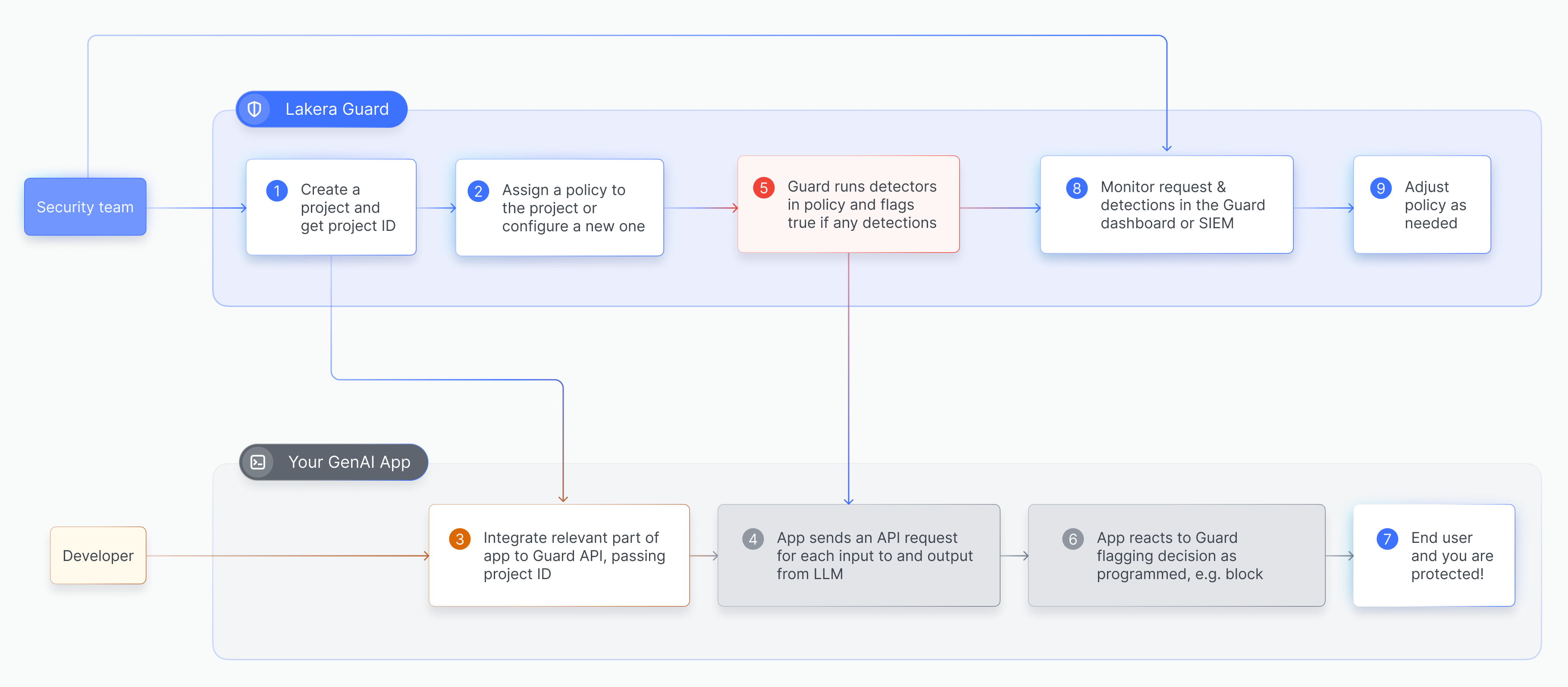
Detectors
The Lakera Guard detectors are organized into four defense categories:
-
Prompt defense - Protection against user prompts, documents or other LLM inputs that contain instructions that override the behavior the developer intended or manipulate the LLM into doing something malicious or leaking sensitive data. These nefarious instructions are called prompt attacks and include jailbreaks, prompt injections, and other malicious or manipulative inputs.
-
Content moderation - Ensure that your applications are not generating harmful or embarrassing content, as well as flagging if your users are trying to use your application to produce offensive content or help with dangerous or illcit activities. Content moderation coverage includes:
- Crime
- Hate
- Profanity
- Sexual content
- Violence
- Weapons
- Custom content moderation detectors to flag any trigger words or phrases
-
Data Leakage Prevention - Safeguard Personally Identifiable Information, prevent system prompt leakage, and avoid costly leakage of sensitive data, ensuring compliance with data protection and privacy regulations. PII coverage inclues:
- Full names
- United States mailing addresses
- Phone numbers
- Email addresses
- Internet Protocol (IP) addresses
- Credit card numbers
- International Bank Account Numbers (IBANs)
- United States Social Security Numbers (SSNs)
-
Unknown links - Prevent attackers manipulating the LLM into displaying malicious or phishing links to your users.
You can read more about Lakera Defenses here.
A policy determines the defences to use when securing LLM interactions, as well as the paranoia level, so you can fine tune to your use cases and risk tolerance.
How policies work
Each project in Guard is assigned to a policy configuration. The policy is
set by selecting the defenses you want Guard to run on every
guard
API request that is tagged with the Project ID for that project.
To give some examples, the policy could be to:
- Check user inputs for prompt attacks or any PII.
- Check LLM outputs for content moderation violations or suspicious links from unexpected domains.
Flagging logic
When the detectors specified in the policy screen a request, if they detect something
they will be marked as having ‘flagged’. If any of the detectors flag, then the
guard
request returns flagged equals true
. If none of the detectors
flag then the guard
request returns flagged equals false
.
You can decide within your applications what to do with a flagged response. You can choose to block the inputs being passed to the LLM or the LLM output returned to the user. You can trigger a confirmation with the user that they want to proceed. You can do nothing and just log it for analysis and monitoring. It’s entirely up to you.
Optionally, a breakdown of the flagging decision can be returned in the response. This will list the detectors that were run, as defined in the policy, and whether each of them detected something or not.
Threshold Levels
You can fine-tune the thresholds for flagging by specifying the confidence level threshold for each defense category. This enables you to fine-tune the strictness and risk threshold to each of your use cases.
For example, if you had a high risk tolerance for one use case you can set a detector to only flag very high confidence detections in order to have low false positives. Or, if there was a use case you wanted to be really sure wasn’t manipulated, even at the cost of reduced user experience, you could set the detector to flag anything that the detector thinks could potentially be a detection.
This is done by setting the confidence level threshold for each defense. This is the level of confidence above which a detector will flag. Lakera Guard uses the following confidence levels:
- L1 - Lenient, very few false positives, if any.
- L2 - Balanced, some false positives.
- L3 - Stricter, expect false positives but very low false negatives.
- L4 - Paranoid, higher false positives but very few false negatives, if any. This is our default confidence threshold.
These levels are in line with OWASP’s paranoia level definitions for WAFs.
Note that the Unknown Links detector cannot be fine-tuned as they
return only a binary match, true
or false
.
The Lakera Guard results
endpoint can be used to get the detector confidence
threshold level results of a screening request. This endpoint can be used to analyse
your data to determine the appropriate threshold for a flagging decision for blocking,
for example, or for ongoing detector performance monitoring. It is not intended for the
results
endpoint to be used in runtime application decisions, as
this prevents them being controlled or modifiable by policy.
Default Policy
Any request tagged with a project ID will have the detectors run as specified in the
policy assigned to the project. If no project_id
is passed in the request, or the
project is not mapped to a custom policy, then the Lakera Guard Default Policy is
used.
This means a new project can already start being protected by Guard before setting up a custom policy, or without having a custom policy at all. It also functions as a fallback option making sure no Guard screening requests can be accidentally sent without any protections.
The Lakera Guard Default Policy screens any content passed with all of the defenses’ detectors (Prompt defense, Content Moderation, PII, Unknown Links), all with the strictest confidence level threshold of L4. This ensures GenAI applications are secure by default.
The Guard API can be used without any projects or policies set up. The Lakera Guard Default Policy will just be used for every request.
Note that the Default Policy will automatically incorporate any new detectors Lakera releases in future.
Latency considerations
The latency of the guard
API response depends on the length of the content passed for
screening, as well as the detectors used for screening according to the policy.
Note that making changes to a policy may have an impact on the latency experienced by your application and end users.
If you need help aligning your policies to meet strict latency requirements please reach out to support@lakera.ai.
Policy Example
To give an example of policies in action, you could set up a policy for your customer support chatbot with the following configuration:
-
Input settings: _ Prompt defense with threshold level L2, meaning any screening requests that Guard is confident or thinks is very likely to be a prompt attack will be flagged. _ PII screening for credit card numbers, IBAN codes and US Social Security Numbers. This is to stop malicious users extracting data, as well as prevent users unwittingly leaking their most important data to the app and third party LLM provider.
-
Output settings:
- All of content moderation with threshold level L3, so Guard flags anything that is at least likely to be moderated content. This is to make sure the chatbot isn’t manipulated into saying anything inappropriate.
- A custom content moderation detector that flags any mention of your main competitor’s brand name or products.
- Unknown links, with your own domain added to the allowed domain list. This prevents the LLM being tricked into sharing phishing or malicious links with users via indirect prompt attack.
Now let’s imagine one of your products is jerk seasoning and you notice that Guard is flagging any mention of this as “jerk” is flagged as potential profanity due to your more aggressive content moderation configuration.
This can be quickly addressed by editing the policy and setting the threshold level for profanity to threshold level L1 and leaving the other content moderation detectors at level L3. This updates Guard straight away and your customers can freely ask your chatbot questions about jerk seasoning!
Setting up and configuring policies
The process for setting up and configuring policies is different depending on your deployment option. Please read the relevant page for details: