Integration and Architecture
After baselining performance metrics, the next step is to determine how well Lakera Guard works in integrated use cases. Lakera Guard functions as a control layer around a model, assistant, or agent(s). Whether deployed as a self-hosted container or leveraged via the SaaS service, Lakera Guard fits seamlessly into a wide range of use cases.
Integrating Lakera Guard
Integrating Lakera Guard is as simple as making an API call. You can send requests using any HTTP client following the basic request format. The flexibility of making API calls enables integration into all architectures. Detailed documentation for making API calls is available for each detection endpoint.
- Prompt Injection
- Detection categories:
Prompt Injections
,Jailbreaks
- Detection categories:
- Content Moderation
- Detection categories:
Hate Speech
,Sexual Content
,Profanity
- Detection categories:
- Personally Identifiable Information
- Detection categories:
Credit Card Numbers
,Phone Numbers
,Email Addresses
,IP Addresses
,Full Names
,Mailing Addresses
,US Social Security Numbers
,International Bank Account Numbers
- Detection categories:
- Unknown Links
- Detection categories:
URLs not in Top 1 Million Popular Domains
,Custom Allow List
- Detection categories:
Designing Control Flows
Lakera Guard returns an API response, making no decisions on your behalf. This provides full control over designing workflows based on tolerated risk thresholds. An example API response contains categorical level information, including a true/false
flag and a confidence float score
.
Example response from the Lakera Guard Prompt Injection API endpoint.
When first integrating Lakera Guard, you can choose to use a non-blocking strategy. This simply means integrating Lakera Guard without creating any flows to block input or output on flagged responses. This approach allows you to monitor Guard’s performance and identify relevant confidence scoring.
Sample Use Case: GenAI Chat Application
Generative chat applications are a popular enterprise use case for Lakera Guard. First, consider the data flow of a chat system that does not leverage security controls for managing model input and output.

In this basic implementation, data flows from the user to the model back to the user. Security is dependent on the model’s ability to handle malicious input and control its own output.
This implementation poses several risks, including malicious prompts such as prompt injections or jailbreaks entering the application. It’s also possible for sensitive data, like PII, to enter the model. Depending on compliance requirements, this may pose additional risks. Additionally, there is concern the model may provide the user with undesirable responses, including hate speech or sexual content. Relying on the foundation model developer to address these risks comprehensively is not optimal. Updates to the model can introduce behavioral changes. There’s also potential for creating lock-in conditions which would make using multiple models or switching providers difficult.
Lakera Guard Implementation
Lakera Guard protects against these risks but is abstracted from the model itself. In the generative chat system, a sample implementation sends user input to the Lakera Guard API before passing the prompt to the model. A common configuration is to send requests to the Prompt Injection and PII detection endpoints to check for malicious inputs and sensitive data leaks, respectively. On the model output, it’s common to check for Content Moderation, PII, and Unknown Links in the model response. Note that all endpoints are available for users to configure on input and output.
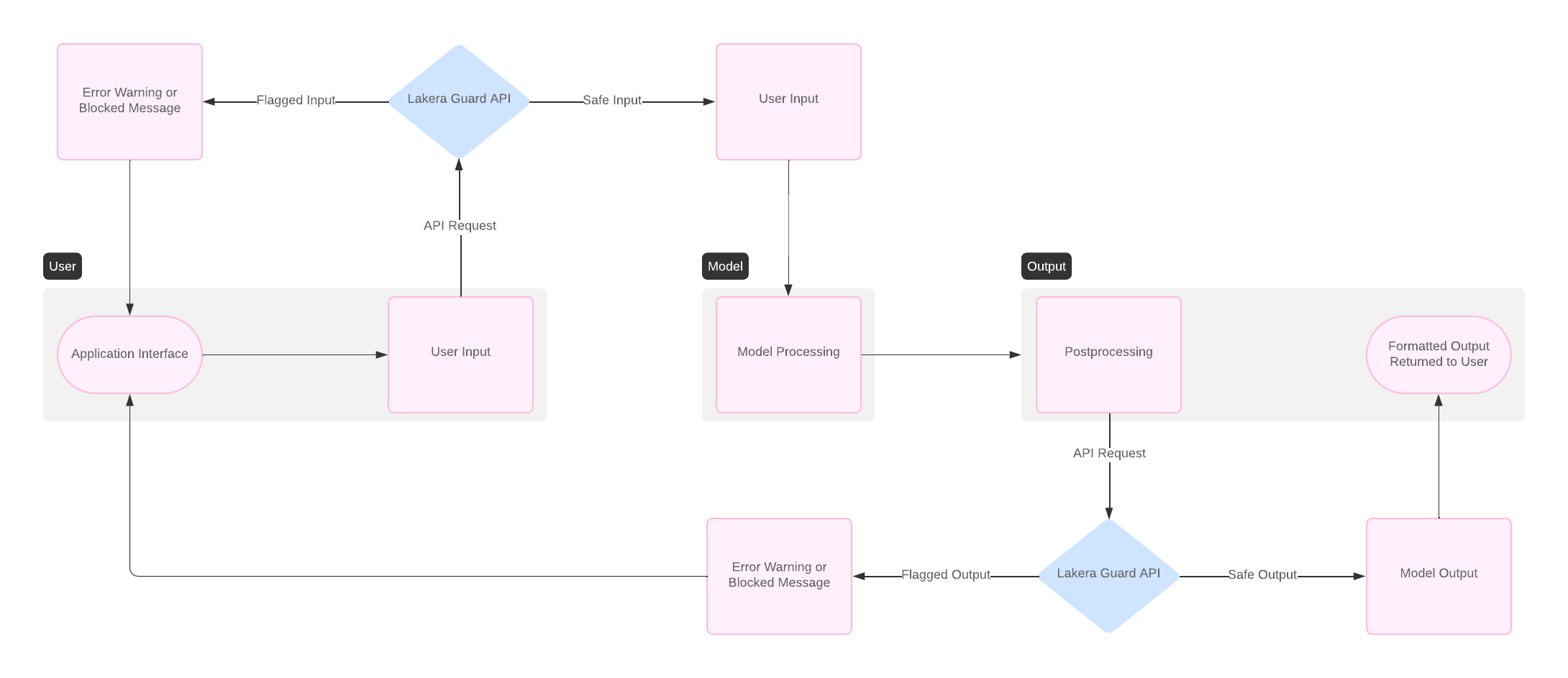
In the diagram above, the GenAI Chat application is secured with Lakera Guard by making an API call containing the user input and an API call containing the model output. In doing so, a control set has been created to enforce what enters and leaves the model without relying on the model itself.
Sample Use Case: Handling Documents
Handling documents with Lakera Guard works similarly. Consider a general data flow of a document entering a model. The first requirement is to handle the document upload and parse it into text. Once parsed, the control flow follows the same structure.
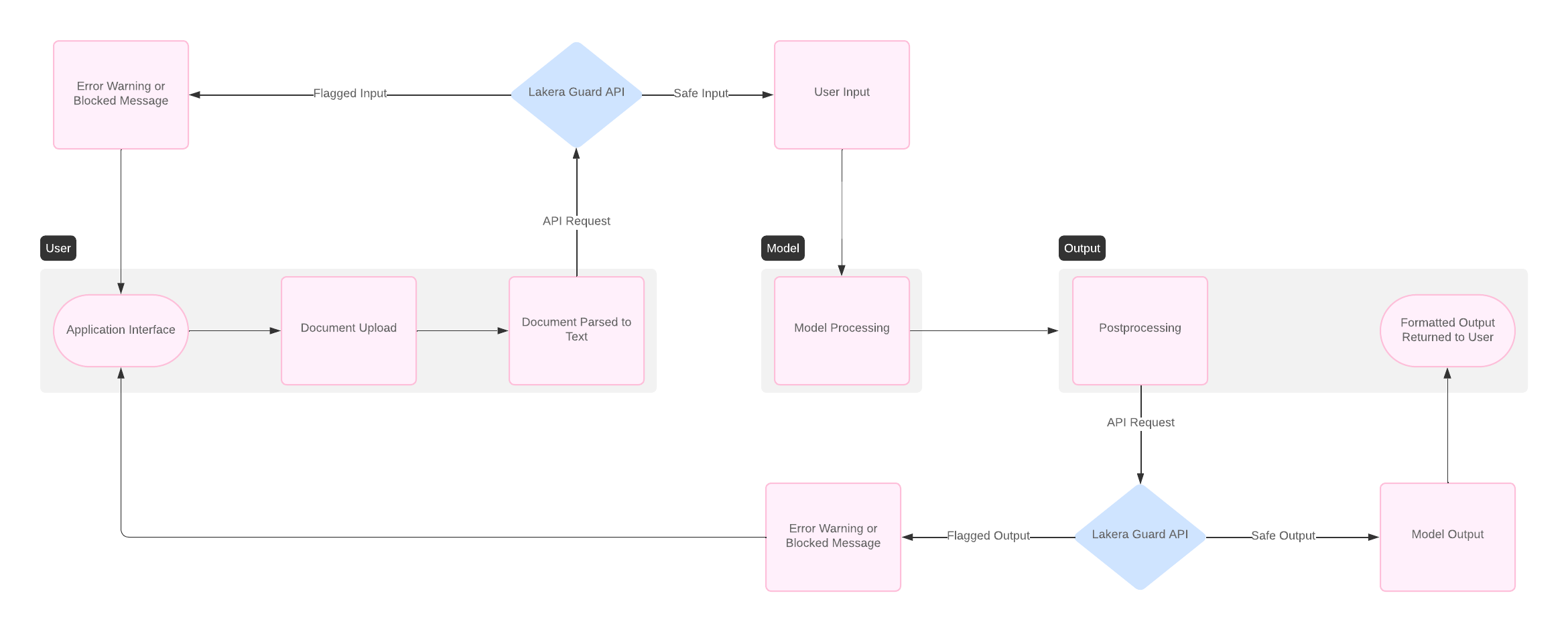
When dealing with large documents, it’s best to parse and chunk the document, sending smaller parallelized requests to Lakera Guard. Baselining latency helps identify the optimal chunking size and performance trade-off.
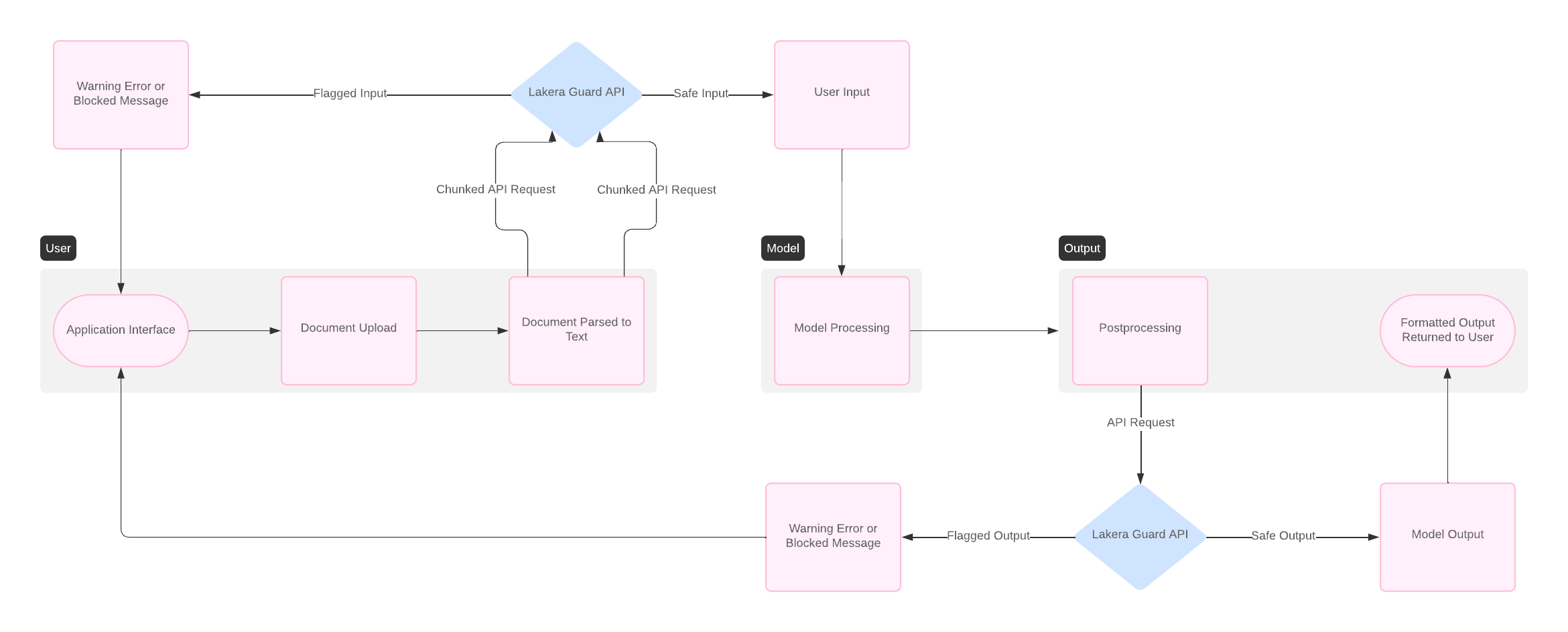
Sample Use Case: RAG Architecture
GenAI Applications with scaled-out knowledge bases can leverage Lakera Guard in the same way, helping to extend protection against documents which may contain poisoned or sensitive data. The following diagram shows a question-answer RAG generation pattern, but is applicable for other RAG use cases.
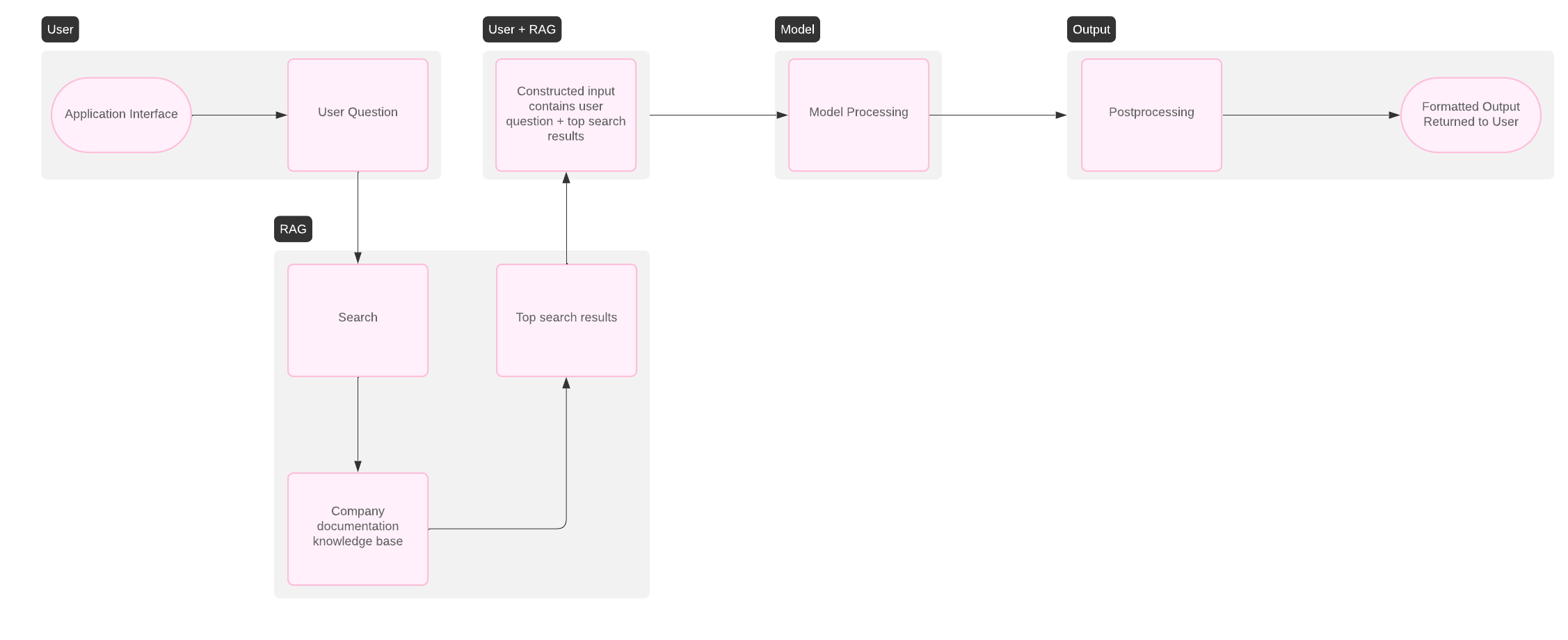
Lakera Guard Implementation
Despite increased architectural complexity, the implementation pattern remains the same. Contained in the API request call to Lakera Guard is the user input and retrieved context. The input is evaluated in its entirety. A common configuration is to detect for Prompt Injection, Content Moderation, Unknown Links, and PII on input. Monitoring the output for Content Moderation, PII, and Unknown Links offers an additional layer of defense-in-depth.
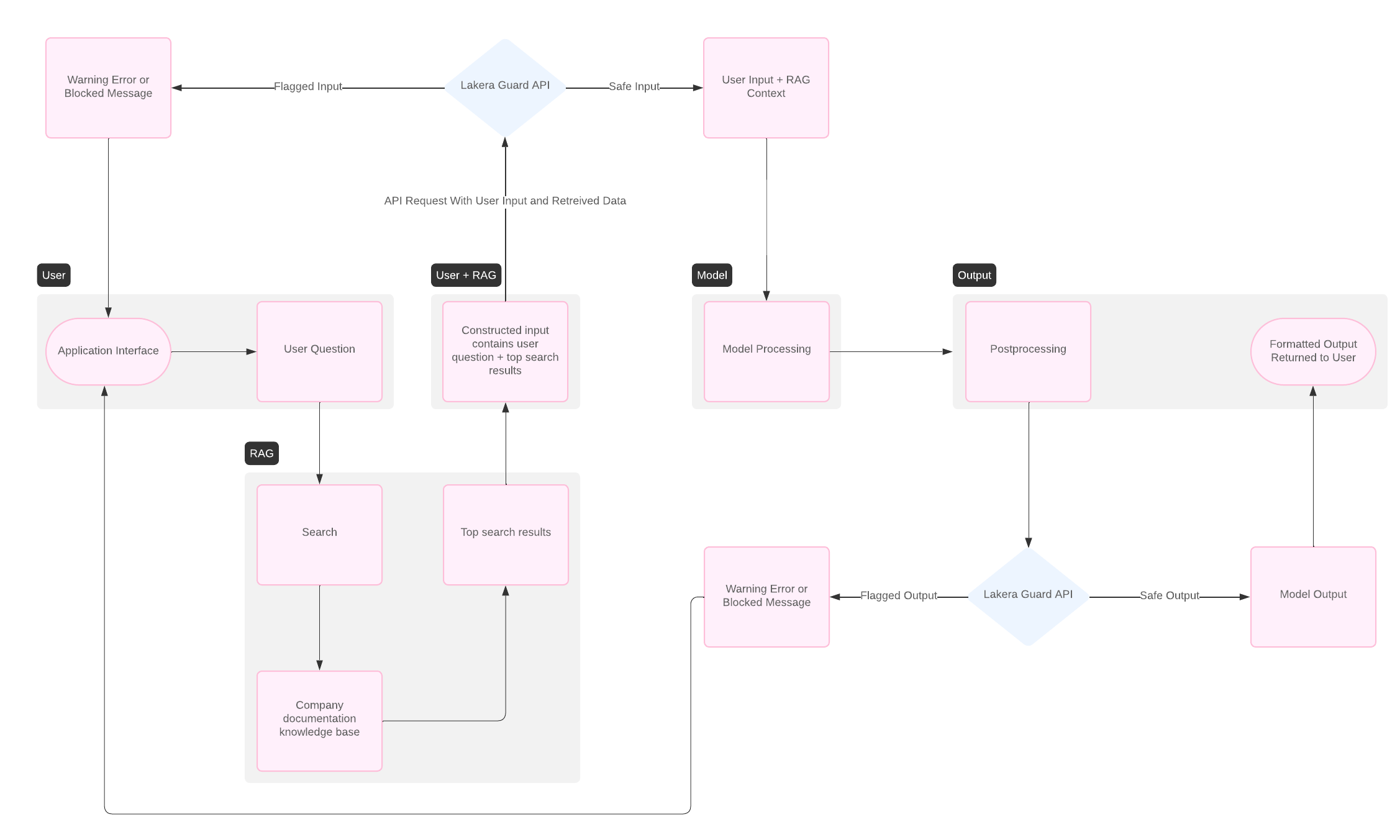
When system prompts are included alongside user inputs and retrieved information, the architecture remains unchanged, but the input requires modification. For optimal use of Lakera Guard with system prompts, it’s recommended to define roles: System
, and User
on input. This approach helps Lakera Guard recognize what input is trusted (the system prompt) and what input is untrusted (the user input and RAG context). Sending the contextualized inputs helps reduce false positives, particularly in cases where system prompts contain imperative language.
Sample Use Case: AI Gateway
Lakera Guard integrates seamlessly with an AI Gateway, providing a centralized access point for managing and securing AI services. This integration ensures consistent control enforcement across all AI interactions. Organizations benefit from this setup through improved efficiency, enhanced observability, and streamlined operations.
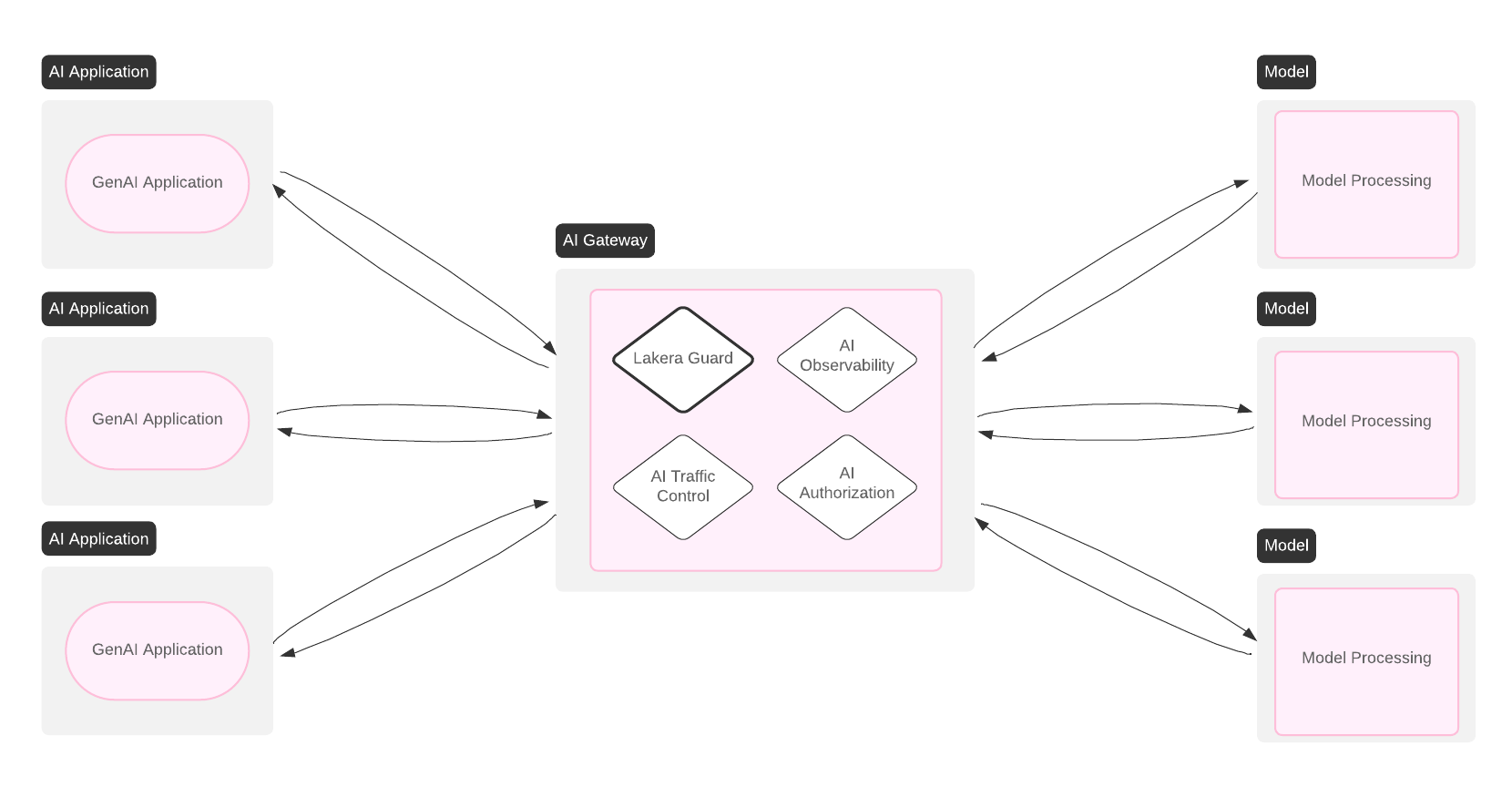